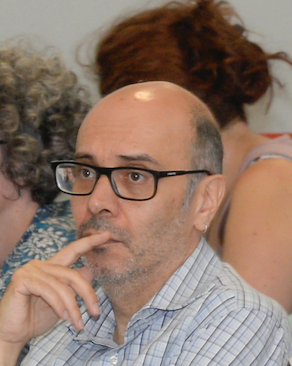
Professore Ordinario, Florence University
Office: 326. Phone: +39 055 457 2327
E-mail: fanelli AT fi.infn.it
Publications: Google Scholar
Research topics: complex systems, stochastic modeling, network theory, machine learning.
Research interests
My research interests fall in the realm of statistical mechanics and non linear physics. As an example we are interested in investigating the role played by finite size corrections in shaping the emerging macrospic pattern in models of population dynamics. Under specific conditions, the microscopic disturbances due to the inherent granularity can amplify as follows a complex resonance mechanism and yield organized spatio-temporal patterns. More specifically, the measured concentration which reflects the distribution of the interacting entities (e.g. chemical species, biomolecules) can oscillate regularly in time and/or display spatially patched profile, collective phenomena which testify on a surprising degree of macroscopic order, as mediated by the stochastic component of the dynamics. Our research is aimed at exploring these effects into details. This task is pursued with reference to specific models of broad theoretical and applied relevance. Applications range from neurosciences to molecular biology. In our group we also apply machine learning methods to a wide range of problems (from biology to material science). Further we seek at developing novel strategies for optimal learning so as to improve on current methods. We recently proposed a novel learning scheme which is anchored on reciprocal space. Following this newly proposed approach, one trains the eigenvalues and the eigenvectors of suitable transfer operators, instead of adjusting the weights in direct space. Interestingly, one can freeze the eigenvectors to reference entries and restrict the learning to the eigenvalues. In doing so one still obtains remarkable performance, in terms of classifications scores, while acting on a considerably smaller set of adjustable parameters.